Linking power plant financial data to energy system operational data with help from Climate Change AI
At the end of 2023, Catalyst wrapped up work funded by a Climate Change AI (CCAI) Innovation Grant using entity matching (record linkage) to connect the energy system financial data reported to the US Federal Energy Regulatory Commission (FERC) and physical energy system data reported to the US Energy Information Administration (EIA). While the data published in FERC Form 1 refers to the same utilities, power plants, and generators that are reported by EIA, these entities lack common IDs to link them. This connection between datasets is necessary to show that retiring certain fossil fuel power plants in favor of renewable energy sources is economically beneficial and technically feasible while still meeting the physical demands of today’s grid. Conducting entity matching to model this connection eliminates the extremely laborious process of sifting through these datasets and performing a manual connection. In collaboration with and support of RMI’s Utility Transition Hub, Catalyst created a small validation dataset of manually linked records, and thus know first hand the tedium of conducting this linkage manually.
Over the course of the grant period we developed the connection of FERC Form 1 plants to EIA data from a one-off module to an integrated analysis maintained and deployed with our nightly PUDL builds. Along the way, we updated our FERC-FERC plant connection (the plant_id_ferc1 column in out_ferc1__yearly_all_plants in the PUDL database), providing a unique plant ID to link FERC plants across all years of reporting. We believe our published output table of connections (out_pudl__yearly_assn_eia_ferc1_plant_parts in the PUDL database) is the only regularly updated, free and open-source connection between the FERC and EIA datasets.
We hope the result enables advocates working to decarbonize our electricity system to more easily bring defensible and data-driven analyses to state-level legislative and regulatory processes. Additionally, we hope that the published matching framework can serve as an open-source example of record linkage for energy datasets and be a model for attempting similar connections with other energy datasets.
Inputs
The data published in FERC Form 1 is messy; reported records correspond to an assortment of generator aggregations (e.g. prime mover, primary fuel source, technology type, plants, or generator units). To create an EIA input that could match the diversity of records reported in FERC Form 1, we created the EIA “plant parts table”. This table contains aggregations of all EIA “plant parts” corresponding to the various granularities appearing in the FERC data.
FERC Input: out_ferc1__yearly_all_plants
EIA Input: out_eia__yearly_plant_parts
Model
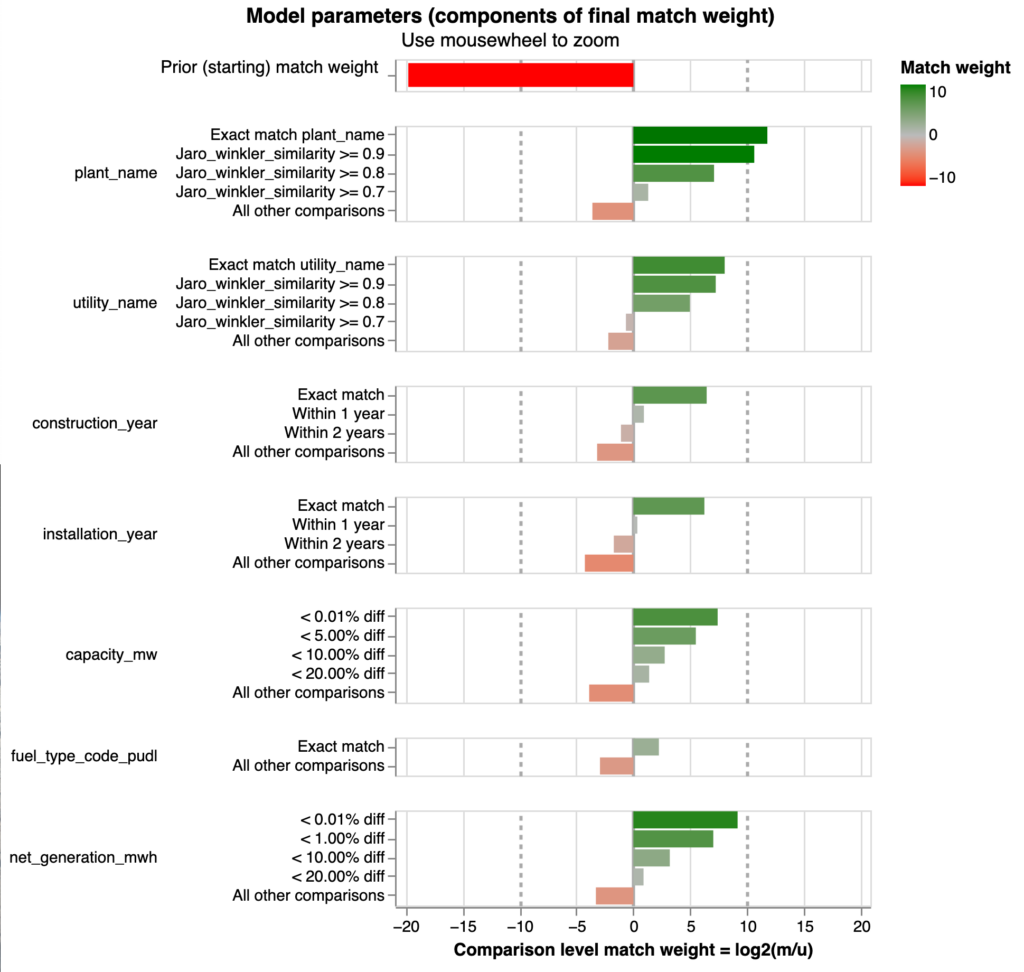
After experimenting with several machine learning packages, we decided to use the open-source Python package Splink as it provided helpful transparency into the effects of changing model parameters and produced results better than our existing baseline. Splink is an entity matching and deduplication interface based on the Fellegi-Sunter algorithm for record linkage. Its main advantages are its speed working with data locally, its interface for users to define fuzzy matching logic between attributes in the input datasets, and its features for doing an unsupervised match (with no training data). Splink provides interactive charts of the model weights that make it easier for downstream users to provide feedback without advanced understanding of the underlying model mechanics.
Results
We used the manually matched dataset to evaluate the model results by a metric of precision and recall. Consider the set of FERC records in this manual validation dataset that the model predicted a matching EIA record for. Precision is the percentage of these matches that are correct. It represents the model’s accuracy when making a prediction. Now, consider all of the FERC records in the manual validation dataset. Recall is the percentage of these FERC records that the model predicted an EIA match for. It represents the model’s coverage of the FERC dataset. The table below displays the precision and recall of the Splink model alongside a baseline linear regression model that was previously integrated into PUDL. The “match probability threshold” is the threshold at which pairs with a lower probability of matching are labeled as a non-match. As the match threshold decreases, more record pairs are labeled as a match and the recall increases. However, precision decreases as the match threshold decreases because the match quality is lower and more FERC records are matched to an incorrect EIA record. Considering the needs of downstream users, we prioritized publishing match results with high precision and thus chose a match threshold of .9 for use in our deployed model.
Match Probability Threshold | Precision | Recall |
.95 | .944 | .833 |
.9 | .943 | .843 |
.75 | .940 | .862 |
.5 | .939 | .875 |
.25 | .938 | .887 |
baseline | .90 | .73 |
Challenges and Limitations
One of the initial challenges we encountered during the project was the high percentage of null values in the input datasets. This significantly impacted the quality of our entity matching results. Additionally, our manually compiled training/validation dataset was relatively small and inherently introduced some unknown biases within the small sample size. Recognizing the dynamic nature of data over time and the potential shifts in representation as more data is published, we additionally experimented with the unsupervised training features for the Splink model. Results were similar to those of the supervised model, and we anticipate using the unsupervised model in the event that the existing training data becomes too outdated or fails to represent evolving patterns in the data. This forward-looking approach ensures adaptability to new data trends and optimizes for scenarios not adequately represented in the initial training dataset.
What’s Next?
With the development of this framework for entity matching, Catalyst is capable of greater flexibility and efficiency in data-driven model development. In 2024, we are building on this framework using funding from the Mozilla Foundation to link Security Exchange Commission utility ownership data to EIA utility operational data. We hope to leverage these models to address analogous issues in natural gas data in the future.
Catalyst is making exciting progress in providing open data to electricity resource planning models like the GridPath RA Toolkit with support from GridLab. Our initial work on these inputs has revealed that there is a need for entity matching in almost all of the datasets under consideration. For example, the Western Electricity Coordinating Council’s Reliability Modeling Anchor Data Set (WECC ADS) has transmission node IDs, generator IDs, and utility IDs that do not match other data sets referring to the same entities. We are excited to utilize the resource efficiency, usability, and transparency of Splink in building entity matching models for these datasets.
Please reach out to us with questions about the modeling process or resulting connection table, and let us know how you are utilizing the FERC to EIA connection!